AI Founder's Bitter Lesson. Chapter 3 - A Footnote in History
I wrote this in December. Right as I was about to publish it, the CEO of Anthropic gave an interview explaining his plan for a “virtual collaborator”. This is a great explanation of what I have called the “horizontal AI product” throughout this series. OpenAI is rumored to be releasing “Operator” within the next few days, which is their version of this. Leaked benchmarks seem to suggest that Operator outperforms Claude’s computer use by a big margin (22% vs 38% on the OSWorld benchmark). This is a big jump, but it’s in line with what I expected 3 months of progress would yield (Claude’s computer use was released in October). I therefore stand by my predictions from December.
Predictions about the future rarely age well, but here we go. The last two chapters showed why vertical AI applications are in trouble: they can’t keep up with more general solutions on performance, and they rarely have a moat to protect their business when horizontal products become competitive. The likely result of this is that there will be a point in time for each vertical where the market begins to switch from vertical to horizontal AI products. But we haven’t answered the most important question: When will this happen? If it takes ten years, it might still make sense to build a vertical app now - but if it happens next year, that’s an entirely different story. This chapter contains my predictions on how the AI application landscape will evolve over the next few years, with specific predictions about the timing of key transitions. Chapter 4 will then explore what this means for founders building in this space.
The change from vertical to horizontal AI products will not happen at the same time in all verticals. Rather, I expect these moments to come in batches with each model release. In some verticals, it might take a long time for this moment to come, but the verticals which most people build today are simple enough so that I expect them to come fairly close together. By 2027, I expect that there will be very few verticals where vertical AI products thrive.
To serve as a table of contents throughout, Figure 2 shows a summary of how I think app adoption will change. I refer to “adoption” as the measure of where people go when they either try to solve a new problem, or change the solution for an existing problem. Note, this measure is:
- Not a measure of market share. Existing deals might lag
- Relative. The pie will grow as AI unlocks more use cases. This is not shown in the diagram.
- Not a measure of potential value. It measures where people go to solve their problems at that point in time, not accounting for future improvements.
For example, the flow from A to B means that a user who used to prefer solution A now would buy solution B to solve their problem.
The terms vertical/horizontal and workflow/agent define different types of AI products. See chapter 1 for these definitions. For simplicity, the diagram combines horizontal agents and workflows into one category. This makes sense because the same companies will likely build both types. For example, ChatGPT might add more agent-like features while keeping its workflow base.
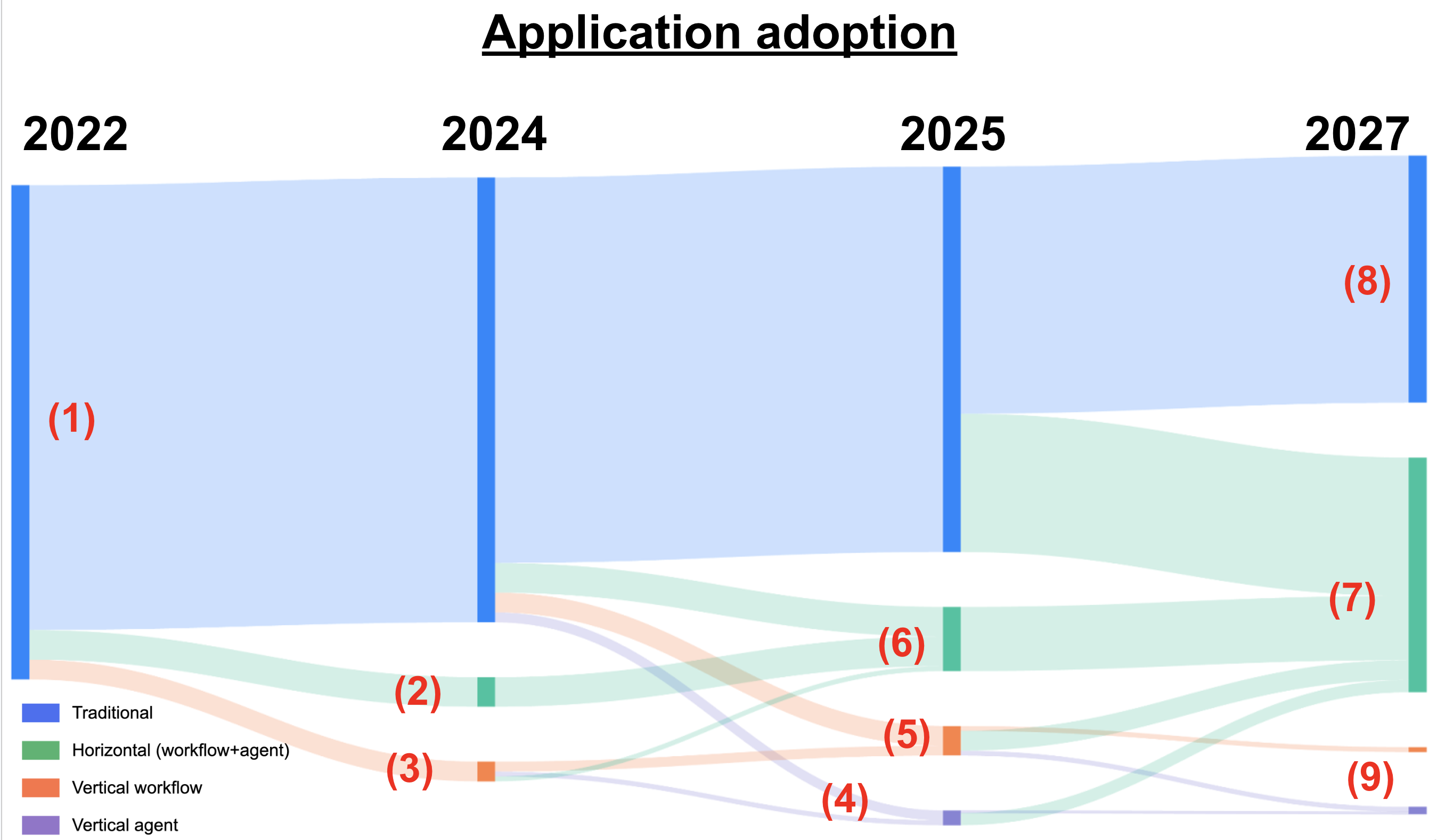
Figure 1: Projected shifts in solution adoption patterns from 2022 to 2027. The diagram shows how user preferences flow between traditional solutions and newer horizontal (workflow + agent) and vertical approaches. The width of each flow indicates relative adoption strength, measured by where users choose to implement new solutions or switch existing ones.
The Past
(1) Pre-ChatGPT era - market dominated by traditional software.
(2) ChatGPT release - the first significant horizontal AI product.
(3) GPT-3.5 API release - first wave of AI verticals.
This year
(4) 2025 will mark a turning point where models become reliable enough for practical agent applications. Until now, agents have existed primarily as research projects or limited proof-of-concepts. While their initial deployment will be modest, their potential impact will become evident. Growth will come from two sources: vertical products upgrading their workflows to agents, and entirely new applications replacing traditional software in ways workflows couldn’t.
(5) Despite agent emergence, vertical workflows will maintain their dominant position through 2025. This persistence stems from two types of switching costs: users’ resistance to changing established tools, and developers’ reluctance to abandon their engineering investments from previous years. The market position these products secured in earlier years creates significant inertia.
(6) The major horizontal AI products (ChatGPT, Claude, and Gemini) will add more features to increase the number of verticals they are useful in. This has already started. For example, ChatGPT now integrates with other desktop apps on your computer. Better models will allow these companies to do this with minimal engineering effort. As these products improve, vertical AI products will find sales harder as people realize that their use-case can be done with a horizontal AI product they already use.
The (near) future
(7). The capability gap between horizontal AI agents and human workers will narrow dramatically. They are not yet at expert level in all domains, but smart enough to reliably handle most tasks which humans typically perform in various traditional software tools. Many humans still keep their jobs, but vertical AI solutions become obsolete. Here are some things I expect this to lead to:
a. Consumers will routinely use horizontal agents for complex tasks like tax preparation, job applications, and non-leisure shopping.
b. Companies will significantly reduce junior-level hiring, with some implementing large-scale layoffs. However, adoption will lag behind theoretical capabilities.
c. We see the first one-person unicorn.
(8) Traditional software will retain value by providing interfaces for agents. While agents could theoretically create the software they need from scratch, computational costs of running the agent make existing software platforms more practical. However, traditional software is not free. I expect that it is the traditional horizontal software that will have the best chance to survive. This is because while agents are not free to run, they are much cheaper than humans. You can, for example, implement a CRM system in excel, but it make sense to buy a specialized CRM system to save a human’s time. But it is not certain that this math checks out for agents.
(9) The only vertical AI applications that survive will be those that locked in a defensible resource, as discussed in Chapter 2. Some will choose to sell their resource for a lot of money.
2024 - has progress stopped?
These predictions assume that AI will continue to improve. We will soon discuss if this is reasonable to expect, but first, let me motivate my choice of the word “continue”. I hear a lot of people claiming that the models have already stagnated. The claim is that we saw no meaningful improvement over GPT-4 in all of 2024. To be fair, I think this narrative has gone more quiet after the release of o3 in December. Figure 2 shows the performance on the famous ARC-AGI benchmark over time. Judge yourself if you think AI improvements have slowed down.
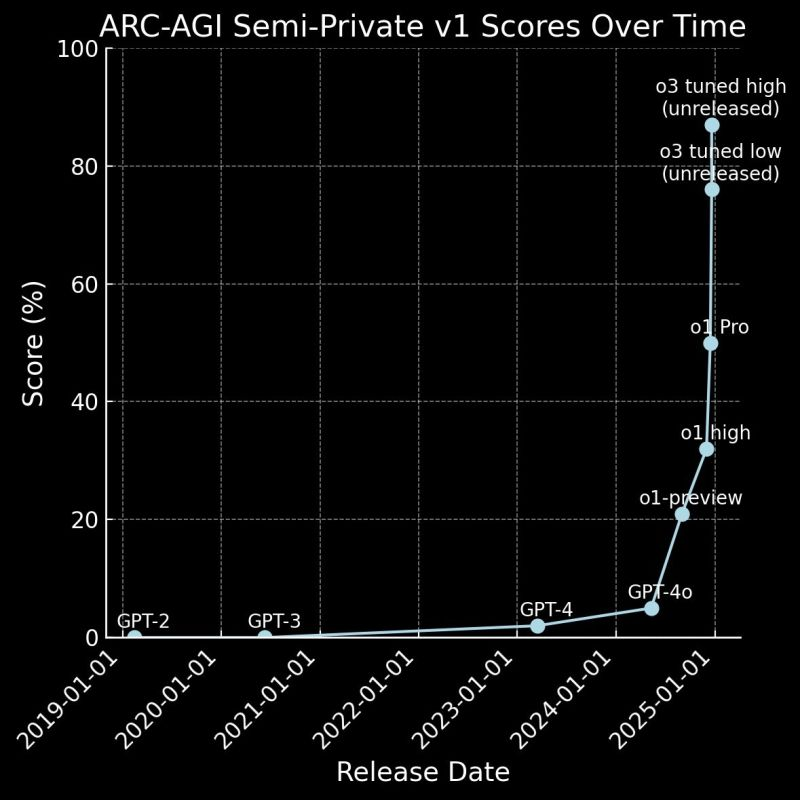
Figure 2: Performance on ARC-AGI benchmark
Even without o3, I still think it is ridiculous to say that models stagnated in 2024. Actually, o3 didn’t update my timeline predictions at all [1]. The zero-to-one moment was o1, which btw, also was released in 2024. But maybe, scaling test time compute does not impress you. After all, high test time compute might be too expensive to use for agents. However, let’s remember what the state of base models were at the start of the year. We had GPT4-turbo which was limited to only text and images. During 2024, OpenAI released GPT4o with audio and video modalities. At the time of the release, it wasn’t a huge intelligence upgrade from GPT4, but since, it has been incrementally upgraded. It is easy to forget how much better it now is.
2024 also saw big improvements for open weight models. On benchmarks with Ph.D-level science questions, we started the year with the best models barely being better than random guessing. By July, we were halfway to human expert level and before the end of the year Deep Seek V3 made an equally sized jump. in 2023 we went from 25-29 (+4), in 2024 29-59 (+20).
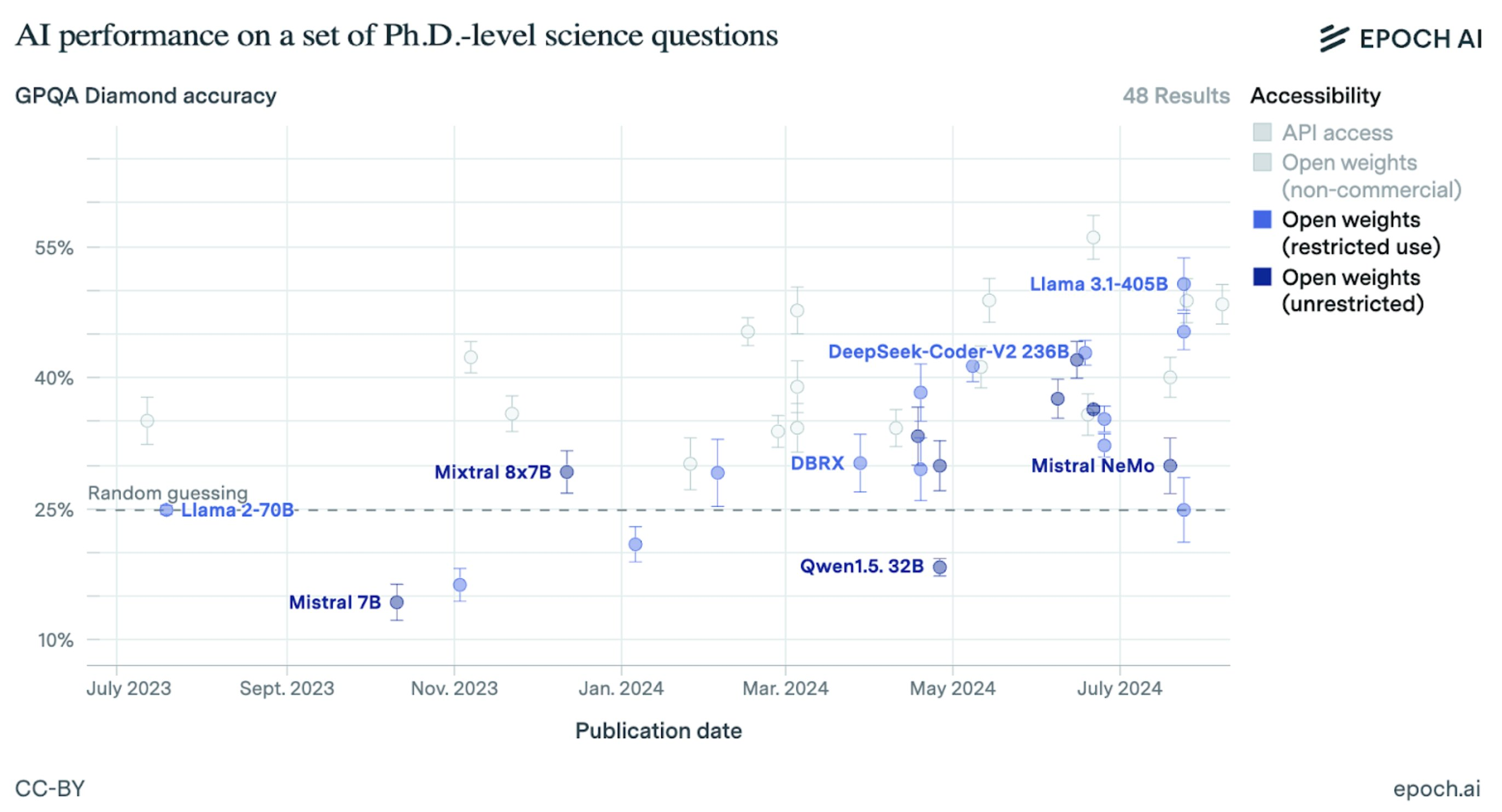
Figure 3: Open weight model performance on GPQA Diamond
However, the biggest contributor of 2024’s improvements was Anthropic. At the start of the year, they had Claude 2 (unusable), in March they released Claude 3 (state of the art), in June they released Claude 3.5 Sonnet (another huge jump). Judging from Figure 4, the spring of 2024 seem to have been the period with the most improvements from base models to date. But what about the fall? Anthropic said that they would release Claude 3.5 Opus by the end of the year, but then quietly removed this from their website. Did the training “fail”? Only Anthropic have the answers here, but many have hypothesized that it didn’t, but that Anthropic saw no economic benefit from publicly releasing it. Instead they used it to generate synthetic data for Claude 3.5 Sonnet. This is supported by the fact that Sonnet saw yet another upgrade in October. This is not what model stagnation looks like.
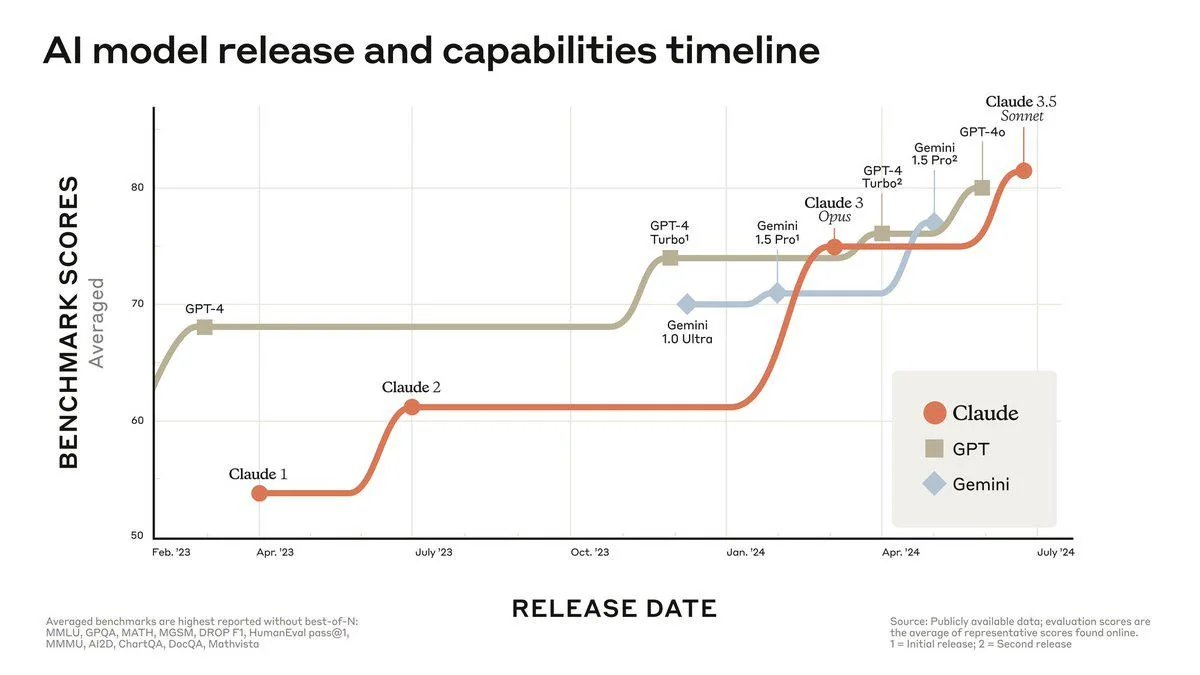
Figure 4: Progress of frontier models on a mix of benchmarks
Potential Roadblocks
While this timeline represents my best guess, several things could change this path. The biggest concerns are:
Model stagnation
2024 was not the year of model stagnation, but maybe 2025 will be? Ilya Sutskever claimed in his NeurIPS talk that scaling pre-training had reached its limits. This got a lot of attention and many interpreted as AI training in general had reached its limits (for example, this article ). However, his claim was only about pre-training. He went on to say that there are other paths to go, for example with test time compute like o1. The subsequent release of o3 solidified the notion that other things than pre-training can work.
Furthermore, as Dylan Patel points out, the leaders in AI development are doubling down on their AI bets by investing more than ever in compute infrastructure. “key decision makers appear to be unwavering in their conviction that scaling laws are alive and well”. Even Yann LeCun, known for being skeptical about language models, seem to have shortened his timelines recently. In December he said that superintelligence is “very far away” but then added “when I say far away, it is not centuries, it may not be decades, but it is several years”].
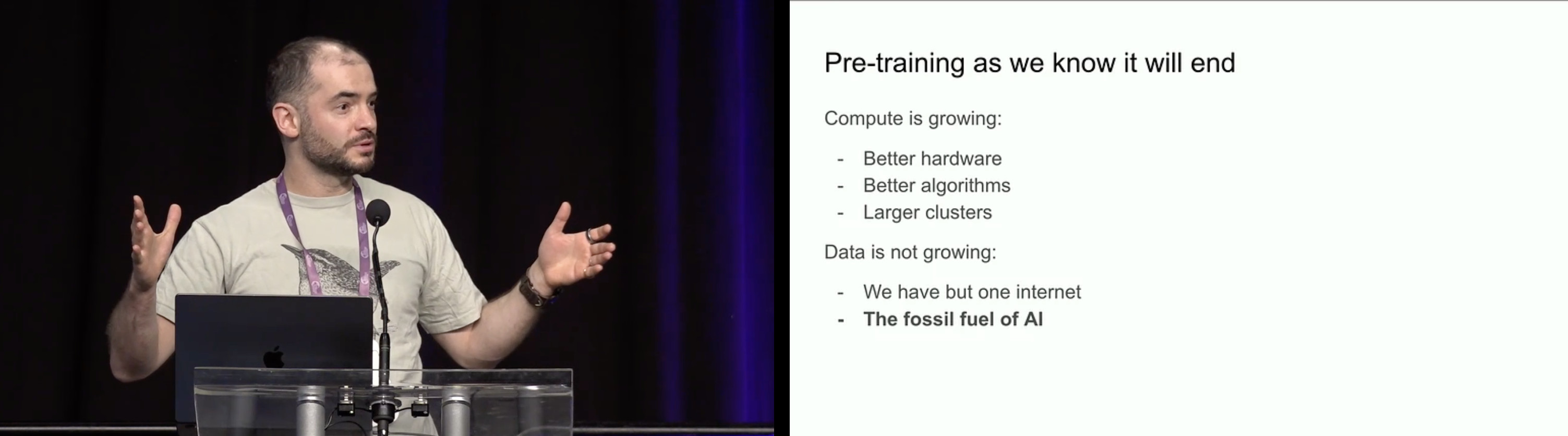
Figure 5: Illya Sutskever’s talk at NeurIPS 2024
Regulation
Current regulatory proposals seem unlikely to slow AI progress significantly (Note: I am not an expert). Most suggested rules have been modest, and even these have struggled to pass. However, a single tragic AI-related incident could quickly shift public opinion and force politicians to take stronger action.
Trust Barriers
People’s current hesitation about AI hallucinations might evolve into broader concerns about letting agents act independently. While I’ve factored some initial resistance into the predictions above, I expect this barrier to fade over time. History offers a useful parallel: people once feared self-driving elevators, an anxiety that seems almost comical today. The adoption pattern for AI agents may follow this familiar path - initial skepticism followed by gradual acceptance as reliability is proven.
AI labs hesitate
The current version of Claude compute use refuses to log in into websites, even if you give it the credentials. Similarly, labs might hesitate to allow the assistant to interact with traditional software in 2027, even if it is technically capable of doing it.
Expensive Inference
OpenAI’s o3 proved that it is possible to spend a lot of money on inference for a single problem and actually get better results. For example, solving problems on the ARC benchmark cost thousands of dollars per task. We might get something similar to Paul Buchheit’s theory, shown in Figure 3. We might achieve the capabilities needed to make a horizontal agent work in every vertical, but find it impractical due to high running costs. However, inference costs have so far dropped steadily. It is also unlikely that the horizontal agent would use max inference compute for every action.
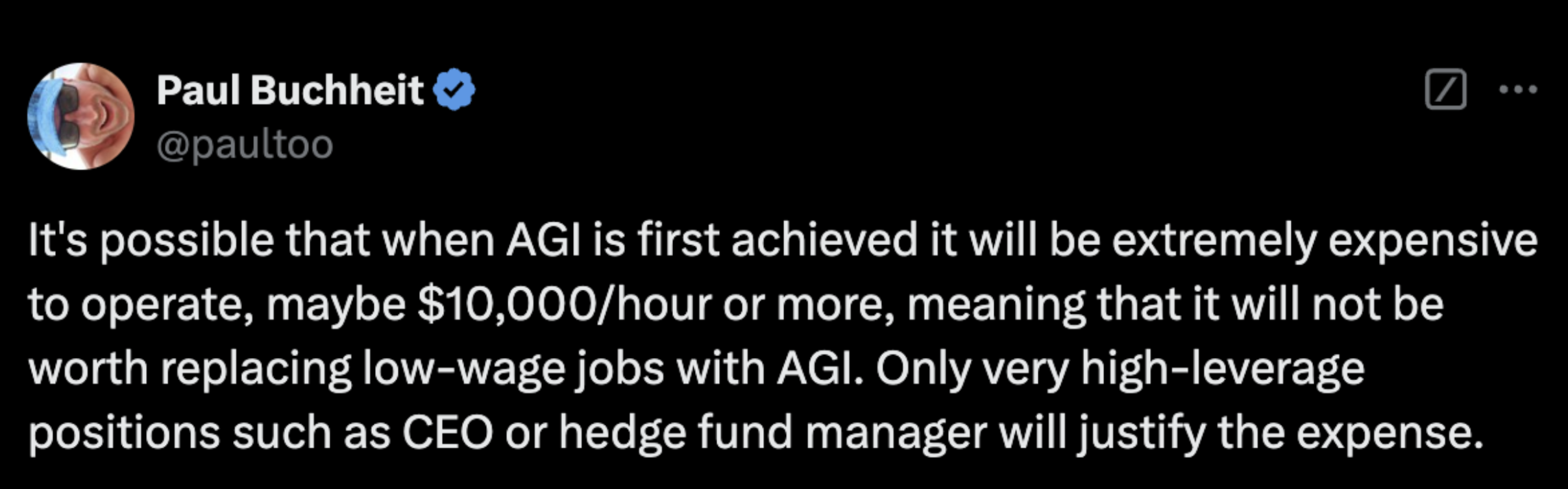
Figure 6: Paul Buchheit’s tweet
Predicting technological change is notoriously difficult, and the roadblocks discussed above could alter this timeline significantly. However, if this trajectory holds, startups in the AI application layer face a challenging situation. They’ll likely struggle to compete with AI labs in building horizontal products, while the window for creating value through vertical applications will be short-lived. As shown in Figure 4, I expect the total value of startups in this space to follow an inverted U-shape curve - rising as engineering effort creates initial value, then falling as better models make that engineering work obsolete.
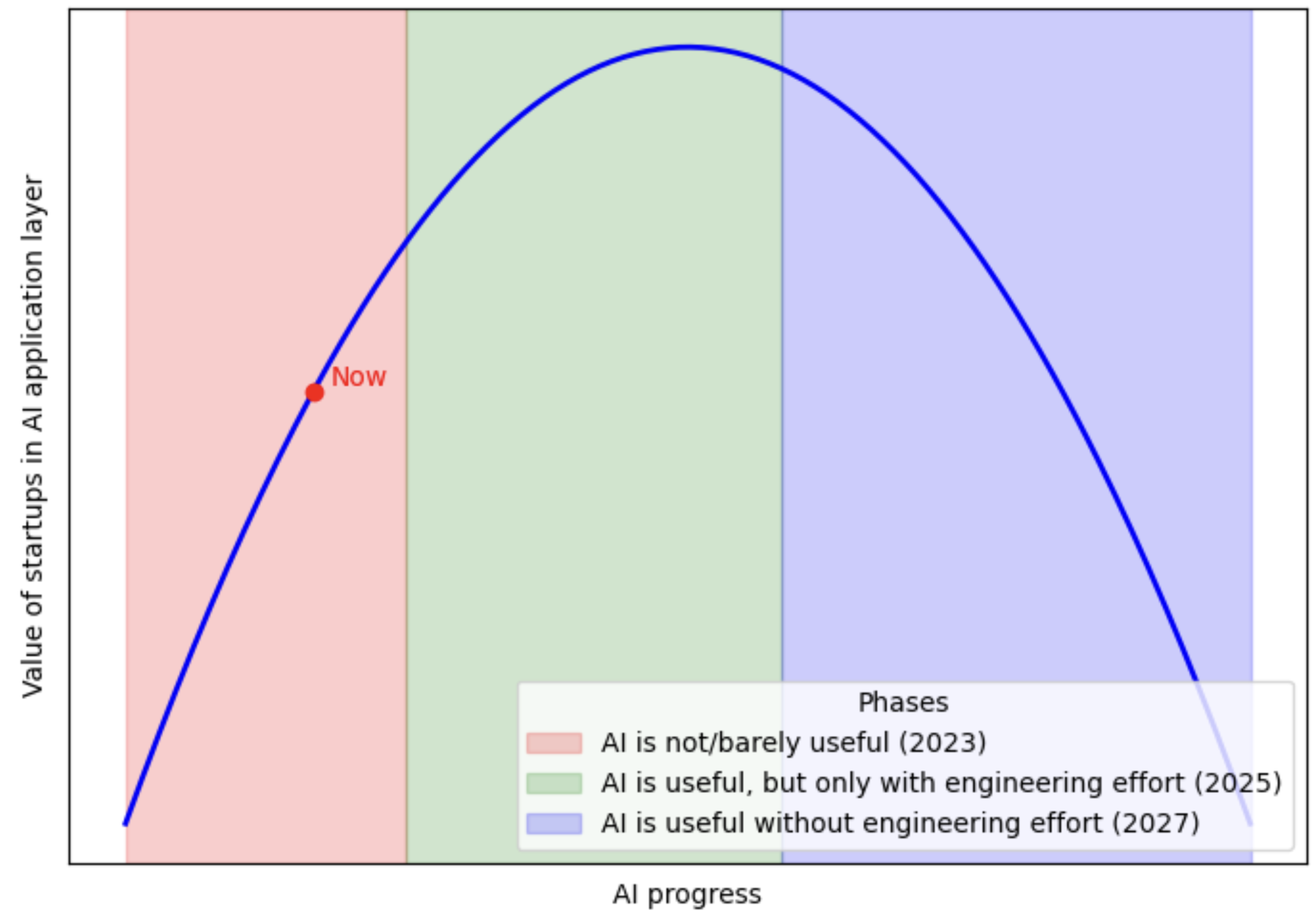
Figure 7: Graph showing the expected value of AI application layer startups over time, divided into three phases.
This might seem discouraging for founders. I got a lot of comments on chapters 1 and 2 along the lines of “so you are saying we should just give up?”, but this is not at all what I am saying. There are plenty of problems out there, an AI app is far from the only thing you can do. For founders considering their next move, there are several questions: Could building a vertical application serve as strategic positioning for future opportunities? If not, what else can I build? Chapter 4 will explore these questions!
Notes:
[1] o3 didn’t update my timeline predictions at all. We knew there were gains to be had from scaling test-time compute. The “Let’s verify step by step” paper proved this in 2023, and o1 showed concretely that it works. When has the first version of a technology ever been the final one? We also know from the AlphaZero series that ML becomes superhuman very quickly in domains with verifiable outcomes. o1 showed that this includes coding and math with an action space of natural language. However, o1 is not better at other domains, such as creative writing. We did not see any indication that o3 is more general than o1.
Thanks to Axel Backlund for the discussions that led to this post.
Enjoy Reading This Article?
Here are some more articles you might like to read next: